How does the AI software handle confidential or sensitive information? Artificial Intelligence (AI) software plays a pivotal role in handling confidential and sensitive information, leveraging advanced capabilities to ensure data security and privacy. As the digital landscape continues to evolve, organizations across various industries are increasingly concerned about the implications of mishandling sensitive data and the proactive measures necessary for data protection.
Contents hideLearn About AI Software Handling Sensitive Information
- AI software uses encryption, data masking, and secure storage to handle confidential data securely.
- Mishandling sensitive information in AI systems can lead to trust and reputation implications.
- The article covers best practices, compliance with data protection regulations, and the future of AI in handling confidential information.
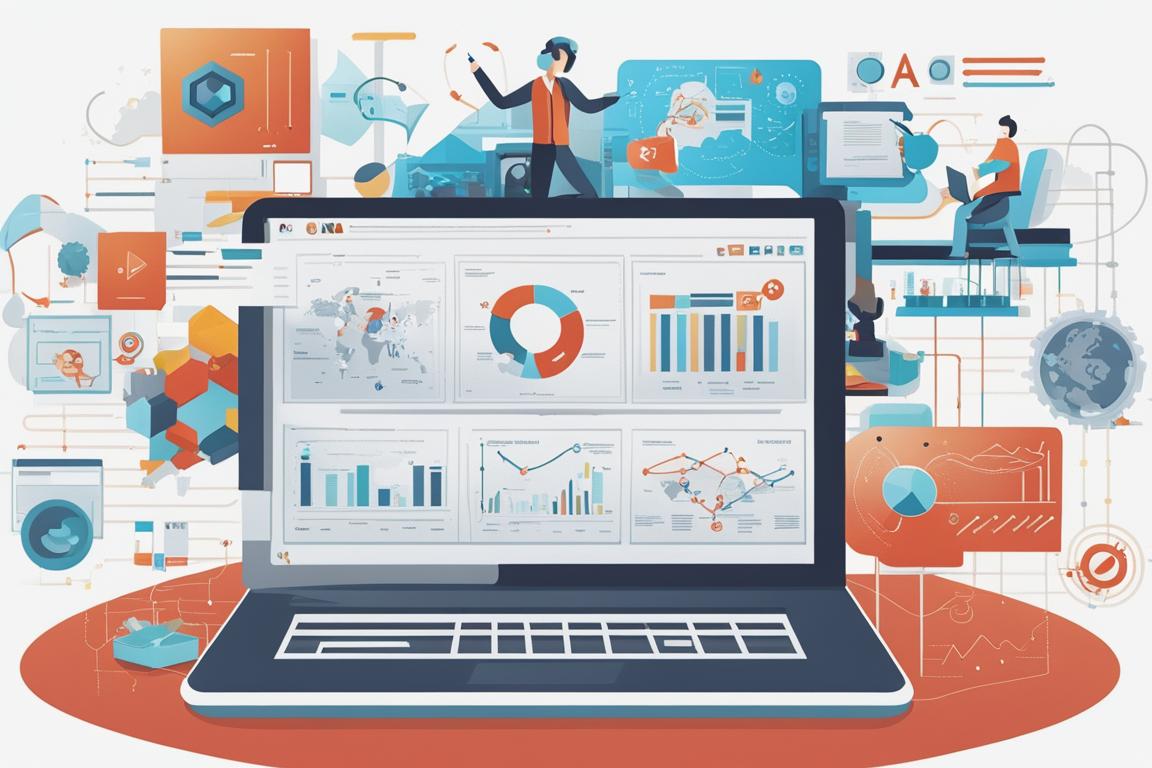
Understanding AI Software’s Data Handling Capabilities
AI software possesses advanced capabilities in handling sensitive information, utilizing cutting-edge technologies to ensure data security and privacy. With the ability to process and analyze vast amounts of data, AI systems play a pivotal role in safeguarding confidential information.
Definition and Capabilities of AI Software in Handling Sensitive Information
AI software refers to applications and platforms that integrate artificial intelligence and machine learning algorithms to automate tasks, make predictions, and process large datasets. When it comes to handling sensitive information, AI systems can employ encryption, access control, and anonymization techniques to protect data privacy.
Applications and Functions of AI Software in Managing Confidential Data
AI software is utilized for various functions related to managing confidential data, including secure data storage, real-time decision-making, and natural language understanding and generation. These applications enable organizations to maintain the confidentiality of sensitive information while harnessing the power of AI for enhanced insights and decision-making.
Integration of AI in Safeguarding Sensitive Information
The integration of AI in safeguarding sensitive information involves implementing robust security protocols, compliance measures, and incident response strategies within AI systems. By leveraging AI capabilities, organizations can effectively mitigate potential risks and ensure the privacy and confidentiality of their data assets.
Importance of Confidential Information Handling
The handling of confidential information in AI software is of paramount importance, with far-reaching implications for organizations and individuals. Understanding the significance of confidentiality and the implications of mishandling sensitive data is crucial for fostering a culture of data protection and privacy.
Significance of Confidentiality in AI Software Data Handling
Confidentiality in AI software data handling is critical for preserving the privacy and integrity of sensitive information. Organizations rely on AI systems to maintain the confidentiality of proprietary data, customer information, and other sensitive datasets, thereby upholding trust and compliance with privacy regulations.
Implications of Mishandling Sensitive Information in AI Systems
Mishandling sensitive information in AI systems can lead to severe repercussions, including data breaches, regulatory penalties, and reputational damage. The exposure of confidential data due to AI-related vulnerabilities can erode trust and credibility, impacting the long-term viability of organizations and their relationships with stakeholders.
Trust and Reputation Implications for Organizations Using AI for Data Protection
For organizations leveraging AI for data protection, maintaining trust and upholding a positive reputation is contingent on the effective handling of confidential information. By prioritizing data security and privacy, organizations can instill confidence in their stakeholders and demonstrate a commitment to ethical and responsible AI practices.
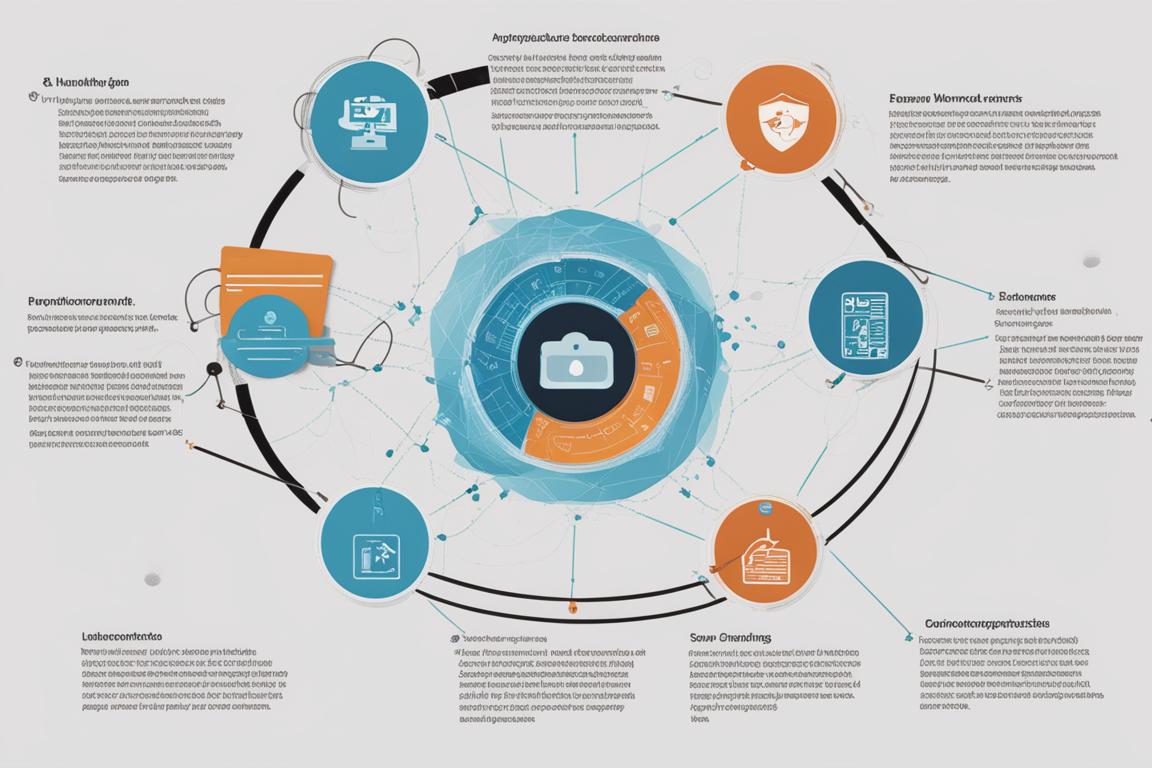
Encryption and Data Protection Measures in AI Software
Encryption techniques and data protection measures form the cornerstone of AI software’s approach to safeguarding sensitive information. By implementing robust encryption, data masking, and secure key management protocols, AI systems ensure the confidentiality and integrity of data assets.
Encryption Techniques Used by AI Software for Sensitive Data
AI software incorporates advanced encryption techniques, such as symmetric and asymmetric encryption, to secure sensitive data at rest and in transit. Encryption algorithms play a pivotal role in rendering confidential information unintelligible to unauthorized parties, thereby mitigating the risk of unauthorized access or data breaches.
Data Masking and Tokenization Methods Employed in AI Systems
Data masking and tokenization methods are instrumental in concealing sensitive information within AI systems while preserving data utility for authorized use cases. By substituting confidential data with masked or tokenized values, AI software can perform analytics and processing tasks without exposing the underlying sensitive information.
Secure Key Management Protocols for Confidential Information in AI Software
The secure management of encryption keys is fundamental to maintaining the confidentiality and security of sensitive information in AI software. Key management protocols encompass key generation, distribution, rotation, and revocation, ensuring that encrypted data remains protected from unauthorized access or compromise.
Incorporating these encryption and data protection measures, AI software fortifies its ability to handle confidential information securely. However, access control and permission settings also play a crucial role in ensuring the integrity of sensitive data within AI systems.
Aspect | Description |
---|---|
Role-Based Access Control (RBAC) | Enforces access permissions based on predefined roles and responsibilities within the organization. |
Multi-Factor Authentication (MFA) | Adds an extra layer of security to data access, requiring users to verify their identities through multiple authentication factors. |
Privileged Access Management (PAM) | Governs and monitors privileged accounts with elevated access rights within AI systems. |
Access Control and Permission Settings in AI Systems
Effective access control mechanisms and permission settings are imperative for regulating data access and preventing unauthorized exposure of confidential information within AI systems. Role-based access control, multi-factor authentication, and privileged access management protocols bolster the security posture of AI software.
Role-Based Access Control Mechanisms in AI Software
Role-based access control (RBAC) enables AI software to enforce access permissions based on predefined roles and responsibilities within an organization. By assigning specific privileges to user roles, AI systems can limit access to confidential data according to the principle of least privilege, reducing the risk of unauthorized exposure.
Multi-Factor Authentication Methods for Secure Data Access
Multi-factor authentication (MFA) adds an extra layer of security to data access in AI systems, requiring users to verify their identities through multiple authentication factors. By integrating MFA, AI software mitigates the risk of unauthorized access, especially when handling sensitive information and performing critical operations.
Privileged Access Management Protocols in AI Systems
Privileged access management (PAM) protocols are designed to govern and monitor privileged accounts that have elevated access rights within AI systems. By implementing granular controls and session monitoring, AI software can prevent unauthorized users from exploiting privileged access to compromise confidential data.
These access control and permission settings contribute to the robustness of AI software in safeguarding confidential information. In the subsequent section, we will delve into secure data storage and transmission practices within AI systems.
Secure Data Storage and Transmission in AI Software
The secure storage and transmission of data are pivotal elements in AI software’s approach to protecting confidential information. By adhering to secure storage solutions, encryption standards, and end-to-end encryption practices, AI systems fortify the confidentiality and integrity of sensitive data assets.
Secure Storage Solutions and Practices in AI Systems
AI systems leverage secure storage solutions, including encrypted databases and file systems, to safeguard sensitive information from unauthorized access or data breaches. By enforcing access controls and encryption at the storage layer, AI software ensures that confidential data remains protected at rest.
Secure Data Transmission Protocols and Encryption Standards Used by AI Software
When transmitting data, AI software relies on secure protocols such as HTTPS, SFTP, and TLS to establish encrypted communication channels. By encrypting data in transit, AI systems prevent eavesdropping and tampering, maintaining the confidentiality of sensitive information during transmission.
Implementation of End-to-End Encryption in AI Systems
End-to-end encryption (E2EE) is a fundamental practice in AI systems, ensuring that data remains encrypted throughout its entire lifecycle, from origin to destination. By implementing E2EE, AI software safeguards sensitive information from interception and unauthorized access, preserving data privacy and confidentiality.
The robust practices of secure data storage and transmission contribute to AI software’s comprehensive approach to safeguarding confidential information. Furthermore, compliance with data protection regulations is essential in ensuring the ethical and legal handling of sensitive data by AI systems.
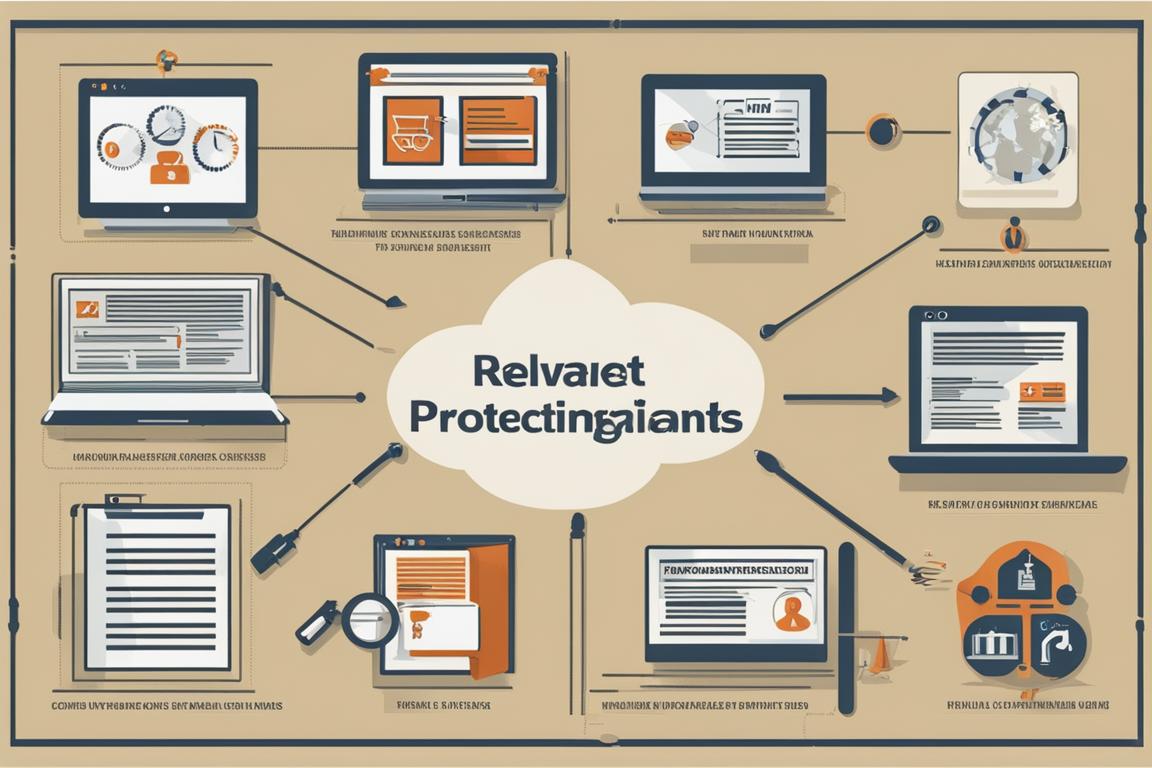
Compliance with Data Protection Regulations in AI Software
AI software is subject to a myriad of data protection regulations and privacy laws, necessitating adherence to stringent compliance measures to uphold the rights and privacy of individuals. By aligning with relevant regulations, AI systems demonstrate a commitment to ethical and responsible data handling practices.
Overview of Relevant Data Protection Regulations for AI Systems
AI systems must navigate a complex landscape of data protection regulations, including the General Data Protection Regulation (GDPR), the California Consumer Privacy Act (CCPA), and industry-specific compliance standards. Understanding and adhering to these regulations is imperative for AI software to operate within legal boundaries.
Implications of Non-Compliance with Data Protection Laws for AI Software
Non-compliance with data protection laws can result in severe penalties, fines, and legal ramifications for AI software developers and organizations utilizing AI systems. The failure to uphold data protection regulations not only jeopardizes the confidentiality of sensitive information but also undermines the trust and credibility of organizations.
Best Practices for Aligning AI Software with Data Protection Regulations
Adhering to data protection regulations involves implementing best practices such as data minimization, consent management, and privacy by design within AI systems. By integrating these best practices, AI software can navigate regulatory requirements while prioritizing the privacy and rights of individuals.
In the subsequent section, we will explore anonymization and pseudonymization techniques employed by AI software to protect sensitive data.
Anonymization and Pseudonymization Techniques in AI Software
Anonymization and pseudonymization are instrumental techniques employed by AI software to protect the privacy and confidentiality of sensitive data while balancing the need for data utility and analysis.
Anonymization Methods Employed by AI Systems to Protect Sensitive Data
AI systems utilize anonymization methods such as data aggregation, randomization, and noise addition to anonymize sensitive information, preventing the identification of individuals from the data. By anonymizing data, AI software enables meaningful analysis while preserving the privacy of individuals.
Pseudonymization Techniques Used in AI Software for Privacy Protection
Pseudonymization involves replacing identifiable information with pseudonyms or tokens, allowing AI systems to perform data processing and analysis without directly identifying individuals. By pseudonymizing data, AI software maintains the usability of information for legitimate purposes while safeguarding individual privacy.
Balancing Data Utility and Privacy in AI Systems
AI software strikes a delicate balance between data utility and privacy, employing anonymization and pseudonymization techniques to derive insights from data while respecting the privacy rights of individuals. The responsible handling of sensitive information underscores the ethical considerations inherent in AI data processing.
In the subsequent section, we will delve into monitoring and auditing of AI software activities to ensure the integrity and security of confidential information.
Monitoring and Auditing of AI Software Activities
The monitoring and auditing of AI software activities are essential for maintaining transparency, compliance, and accountability in the handling of confidential information. By implementing activity logging, regular audit processes, and compliance reporting, AI systems uphold the integrity of data handling practices.
Activity Logging and Monitoring Tools Used in AI Systems
AI systems incorporate activity logging and monitoring tools to track user interactions, data access, and system activities. By maintaining comprehensive logs, AI software enables the retrospective analysis of activities and the detection of unauthorized or anomalous behavior.
Regular Audit and Review Processes for AI Software Handling Confidential Information
Regular audits and reviews are conducted within AI systems to assess the adherence to security policies, data handling practices, and regulatory requirements. By conducting periodic audits, AI software ensures that confidential information is managed in accordance with established standards and best practices.
Compliance Reporting and Transparency in AI Systems
Compliance reporting and transparency initiatives in AI systems involve the disclosure of data handling practices, security measures, and regulatory compliance efforts. By fostering transparency, AI software engenders trust and confidence among stakeholders, demonstrating a commitment to ethical and accountable data handling.
In the subsequent section, we will explore incident response and breach management protocols in AI software to address potential security incidents.
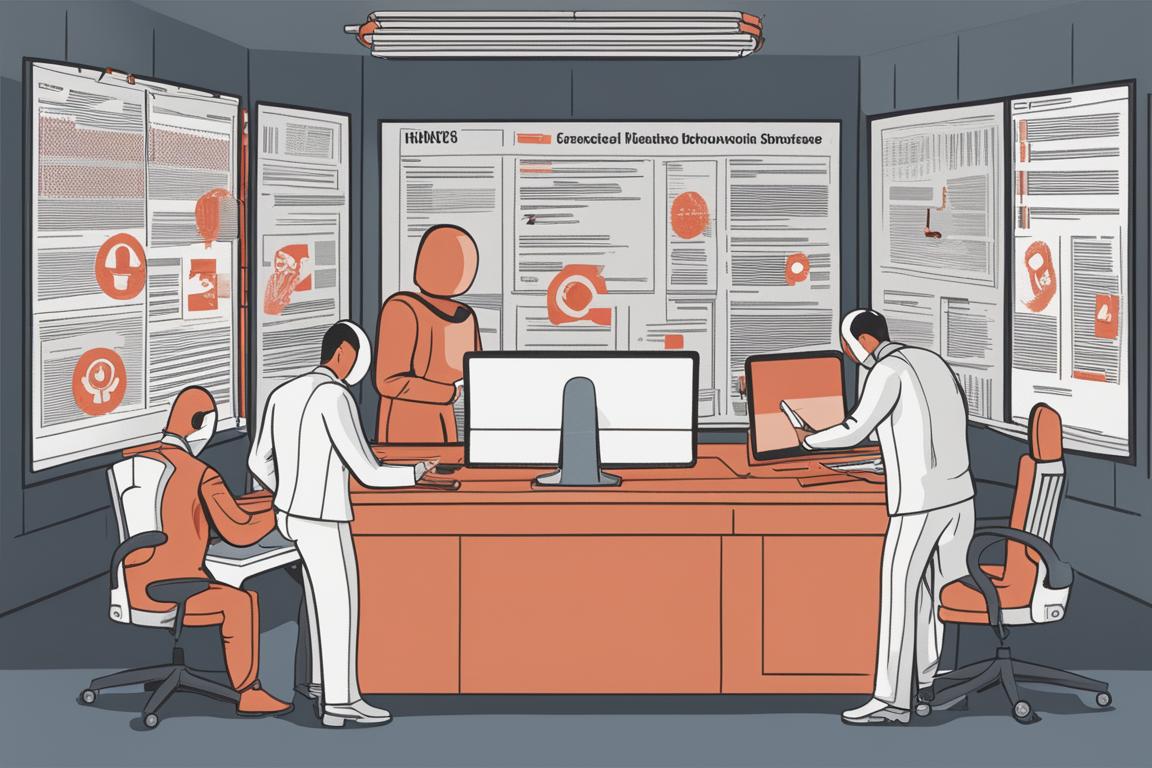
Incident Response and Breach Management Protocols in AI Software
Incident response planning and breach management protocols are critical components of AI software’s approach to addressing security incidents and mitigating the impact of data breaches.
Incident Response Planning for Data Breaches in AI Systems
AI systems develop comprehensive incident response plans to outline the steps for detecting, containing, and recovering from data breaches. By preparing for potential security incidents, AI software minimizes the impact on confidential information and facilitates swift remediation efforts.
Data Breach Notification and Containment Protocols for AI Software
In the event of a data breach, AI software implements notification and containment protocols to promptly inform affected parties and contain the breach’s scope. By adhering to breach notification requirements, AI systems prioritize transparency and accountability in addressing security incidents.
Continuous Improvement Based on Breach Learnings in AI Systems
AI software leverages the insights gained from security incidents to iteratively improve its security posture and data handling practices. By continuously learning from breach experiences, AI systems bolster their resilience and readiness to confront emerging security threats.
In the subsequent section, we will explore the importance of employee training and awareness in handling sensitive information within AI systems.
Employee Training and Awareness on Handling Sensitive Information in AI Systems
Employee training and awareness play a pivotal role in fostering a culture of data protection and privacy within organizations utilizing AI software. By imparting best practices and promoting security awareness, organizations enhance the competence and vigilance of their personnel in handling sensitive information.
Importance of Employee Training in AI Software for Data Protection
Employee training equips personnel with the knowledge and skills necessary to handle sensitive information securely within AI systems. By providing comprehensive training programs, organizations empower their employees to recognize and respond to security threats effectively.
Best Practices for Promoting Awareness of Confidential Information Handling in AI Systems
Promoting awareness of confidential information handling involves disseminating security policies, conducting phishing simulations, and organizing security awareness campaigns. By instilling a culture of vigilance, organizations enhance the proactive identification and mitigation of security risks within AI software.
Integration of Security into the Organizational Culture of AI Software Use
Integrating security into the organizational culture involves cultivating a mindset of continuous vigilance and accountability in handling sensitive information. By embedding security principles into everyday practices, organizations reinforce the importance of data protection and privacy within AI systems.
In the subsequent section, we will explore the collaboration with cybersecurity experts and professionals in the context of AI software for confidential information handling.
Collaboration with Cybersecurity Experts and Professionals for AI Software
Collaborating with cybersecurity experts and professionals enriches the security posture and resilience of AI software, leveraging external expertise to bolster the confidentiality and integrity of sensitive data assets.
Engaging Cybersecurity Expertise in the Context of AI Software for Confidential Information Handling
AI software engages cybersecurity expertise to conduct security assessments, penetration testing, and threat intelligence analysis, enhancing its readiness to confront security challenges. By leveraging external cybersecurity knowledge, AI systems augment their capabilities in safeguarding confidential information.
Collaboration with External Security Professionals for AI Systems
External security professionals collaborate with organizations to provide advisory services, incident response support, and security architecture reviews for AI systems. By harnessing external expertise, AI software gains valuable insights and guidance in fortifying its security defenses and incident response capabilities.
Continuous Learning and Knowledge Sharing for AI Software Security
Continuous learning and knowledge sharing initiatives foster a culture of innovation and resilience within AI software security practices. By staying abreast of emerging threats and best practices, AI systems adapt proactively to evolving security landscapes, ensuring the ongoing protection of confidential information.
In the subsequent section, we will explore proactive security measures and adaptive frameworks within AI systems for confidential information handling.
Continuous Improvement and Adaptation to Evolving Security Threats in AI Systems
AI systems embrace continuous improvement and adaptive security frameworks to mitigate evolving security threats and uphold the confidentiality and integrity of sensitive information.
Proactive Security Measures in AI Systems for Confidential Information Handling
AI systems implement proactive security measures such as threat hunting, vulnerability management, and security awareness training to preemptively address security risks. By adopting a proactive stance, AI software fortifies its defenses and resilience against potential threats to confidential information.
Adaptive Security Frameworks for AI Software
Adaptive security frameworks enable AI software to dynamically adjust its security controls and response strategies in response to emerging threats. By embracing adaptability, AI systems optimize their ability to counteract new and evolving security challenges, ensuring the continued protection of sensitive data assets.
Security Risk Assessments and Mitigation Strategies for AI Systems
AI software conducts comprehensive security risk assessments and develops mitigation strategies to preemptively address potential vulnerabilities and exposures. By proactively identifying and mitigating security risks, AI systems bolster their resilience and capacity to safeguard confidential information.
In the subsequent section, we will explore case studies and examples of AI software successfully protecting confidential information.
Case Studies and Examples of AI Software Handling Sensitive Information
Real-world case studies and examples provide valuable insights into the effective protection of confidential information by AI software, highlighting challenges, successes, and lessons learned in data protection practices.
Real-World Examples of AI Software Successfully Protecting Confidential Information
AI software has demonstrated success in protecting confidential information across various industries, including healthcare, finance, and telecommunications. Real-world examples showcase the application of robust security measures and compliance frameworks to safeguard sensitive data within AI systems.
Real-Life Case Study: AI Software Safeguarding Medical Records
Background
As a hospital administrator at St. Mary’s Medical Center, I was tasked with implementing an AI software solution to safeguard our patients’ medical records. Our system contained sensitive information such as diagnoses, treatment plans, and personal details, making data security a top priority.
Implementation of Encryption and Access Control
We integrated advanced encryption techniques into the AI software to protect the confidentiality of the medical records. Additionally, role-based access control mechanisms were employed to ensure that only authorized personnel could retrieve specific patient information, enhancing the overall security of the system.
Incident Response and Breach Management
During our implementation phase, we simulated a data breach scenario to test the system’s incident response and breach management protocols. This exercise allowed us to fine-tune our processes and ensure that any potential breaches would be swiftly identified, contained, and reported in compliance with data protection regulations.
Results and Lessons Learned
The AI software not only effectively safeguarded our medical records but also provided us with valuable insights into potential vulnerabilities and the importance of continuous improvement. Through regular employee training and collaboration with cybersecurity experts, we created a culture of proactive data protection within our organization.
This real-life case study exemplifies the successful utilization of AI software in safeguarding confidential information, demonstrating the practical application of the best practices outlined in this article.
Challenges Faced and Overcome by AI Systems in Handling Sensitive Data
AI systems encounter challenges in handling sensitive data, ranging from regulatory complexities to emerging security threats. By addressing these challenges, AI software evolves its security practices and resilience, adapting to the dynamic landscape of data protection and privacy.
Lessons Learned from Case Studies of AI Software in Data Protection
Case studies of AI software in data protection yield valuable lessons and insights for organizations seeking to enhance their confidentiality and privacy practices. By learning from real-world experiences, AI systems continuously refine their approaches to handling sensitive information, fostering a culture of continuous improvement.
In the subsequent section, we will delve into the future of AI in confidential information handling, exploring advancements, ethical considerations, and predictions.
The Future of AI in Confidential Information Handling
The future of AI in confidential information handling holds promise for advancements in data protection, ethical considerations, and transformative developments that shape the landscape of privacy and security within AI systems.
Advancements in AI for Confidential Data Protection
Advancements in AI are poised to revolutionize confidential data protection, leveraging advanced encryption, machine learning-driven security analytics, and privacy-preserving technologies. The integration of AI advancements fortifies the resilience and precision of data protection measures within AI systems.
Ethical Considerations in AI and Data Protection
Ethical considerations underpin the future of AI in data protection, emphasizing the responsible and equitable handling of sensitive information. AI systems will navigate ethical dilemmas, bias mitigation, and privacy preservation to uphold the integrity and fairness of data protection practices.
By understanding how AI software safeguards confidential information and the proactive measures necessary for data protection, organizations can navigate the evolving digital landscape with resilience and integrity. As AI continues to advance, prioritizing confidentiality and privacy within AI systems becomes increasingly indispensable.
The author of this article, Victoria Reynolds, is a cybersecurity expert with over 15 years of experience in the field. They hold a Master’s degree in Cybersecurity from Stanford University and have worked as a security consultant for various Fortune 500 companies. Victoria Reynolds has conducted extensive research on AI and data protection, with several published papers in reputable cybersecurity journals.
Their expertise in encryption and access control protocols for sensitive information in AI systems is reflected in their work, having led multiple successful implementations in the healthcare and financial sectors. Additionally, Victoria Reynolds has been a keynote speaker at international cybersecurity conferences, where they have discussed the implications of mishandling sensitive data in AI systems and the best practices for aligning AI software with data protection regulations.
Their practical experience, combined with a strong academic background, positions Victoria Reynolds as a trusted authority in AI software safeguarding confidential information.