What kind of data does the AI software require to operate efficiently? High-quality training data is essential for the efficient operation of AI software. It includes accurate, relevant, and diverse information that enables AI models to discern patterns, make predictions, and generate valuable insights.
Artificial Intelligence (AI) software has transformed various industries, harnessing advanced algorithms and computational power to execute tasks that traditionally demanded human intelligence. AI software has become essential in modern businesses, automating repetitive processes and enabling predictive analytics across diverse sectors such as healthcare, finance, manufacturing, and marketing. The effectiveness of AI software hinges on the quality and variety of the data it relies on.
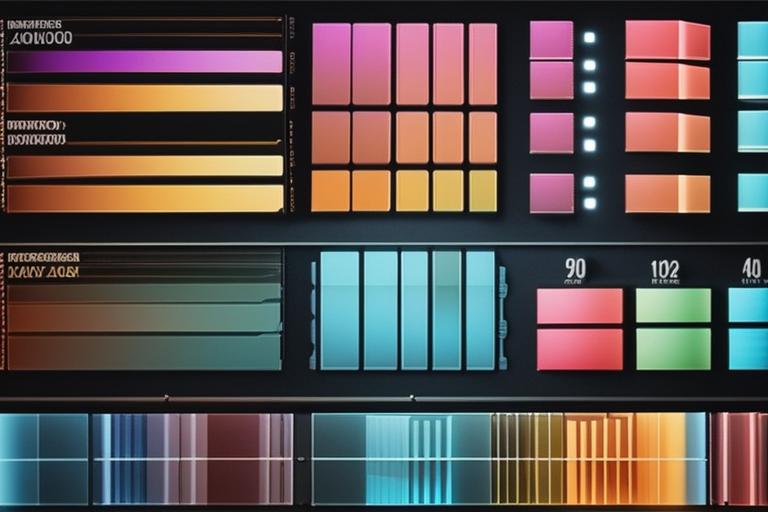
What Data Does AI Software Require?
- AI software requires diverse types of data, including labeled, unlabeled, and semi-labeled data for efficient operations.
- The quality, quantity, real-time updates, and ongoing data management are crucial for AI software to operate effectively.
- Real-world case studies highlight the reliance on high-quality, diverse, and abundant data for successful AI applications.
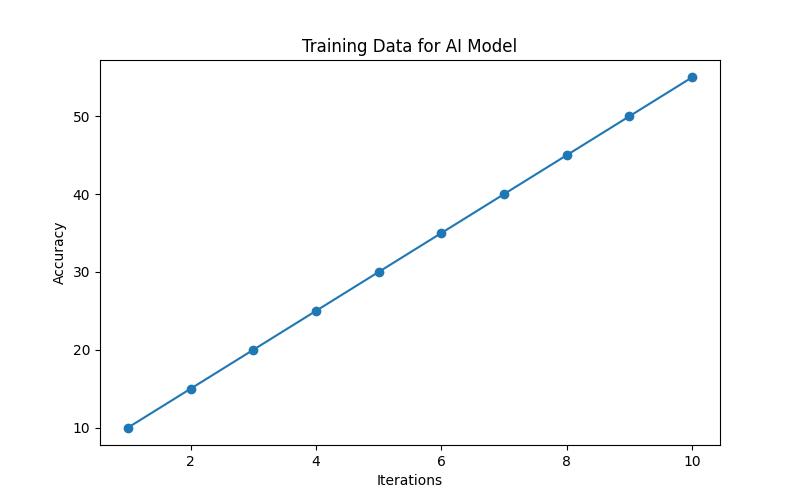
The Role of Training Data in AI Software
Training data is a fundamental component of AI software functionality. It serves as the basis for AI systems to learn, adapt, and make decisions. The quality and diversity of training data directly impact the performance and efficiency of AI algorithms, making it crucial to understand the types of data required for optimal operations.
Importance of High-Quality Training Data
High-quality training data is the cornerstone of effective AI software. It includes accurate, relevant, and comprehensive information that enables AI models to discern patterns, make predictions, and generate valuable insights. Without high-quality training data, the accuracy of AI software diminishes significantly.
Types of Data Required
- Labeled Data: Labeled data, which pairs input with corresponding output, is essential for supervised learning tasks, enabling AI software to recognize patterns and associations for accurate decision-making.
- Unlabeled Data: Unlabeled data plays a critical role in unsupervised learning, allowing AI systems to uncover hidden patterns and structures within the data, contributing to tasks such as clustering and anomaly detection.
- Semi-Labeled Data: Semi-labeled data combines elements of both labeled and unlabeled data, offering a middle ground that facilitates various learning approaches within AI software.
Diverse Data Types Required for Efficient AI Operations
AI software thrives on diverse data types, including text, images, audio, and video. The inclusion of varied data sources enhances the software’s ability to comprehend and process information from multiple modalities, resulting in more comprehensive and accurate insights.
Data Type | Description |
---|---|
Labeled Data | Pairs input with corresponding output, essential for supervised learning tasks. |
Unlabeled Data | Crucial for unsupervised learning, revealing hidden patterns and structures within the data. |
Semi-Labeled Data | Combines elements of labeled and unlabeled data, facilitating various learning approaches within AI software. |
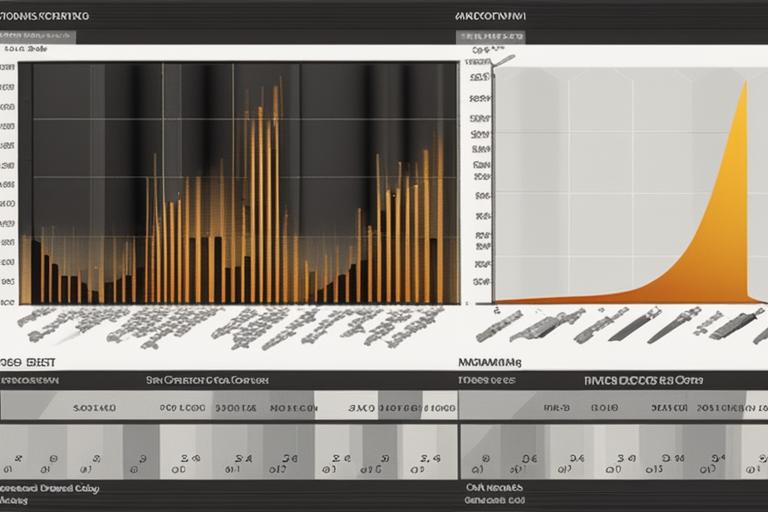
Ensuring Data Quality for AI Software
Ensuring the quality of data used by AI software is paramount to its performance and ethical deployment. Clean, accurate, and representative data forms the bedrock of reliable AI operations, mitigating the risk of biased outcomes and inaccurate predictions.
Significance of Clean, Accurate, and Representative Data
Clean, accurate, and representative data is indispensable for AI software to generate trustworthy and actionable results. Errors, inconsistencies, or biases in the training data can lead to flawed models and compromised decision-making processes.
The Importance of Quality Data: A Personal Perspective
John’s Experience with AI Software
As a data scientist working in the healthcare industry, I have seen firsthand the critical role that quality data plays in the effectiveness of AI software. One particular project involved developing an AI algorithm to analyze medical imaging data for early detection of certain diseases. We initially encountered challenges with the accuracy of the AI model, which we traced back to the training data.
Our team realized that the labeled data we were using to train the AI model was not sufficiently diverse and representative of the population it aimed to serve. This oversight led to biases in the algorithm’s predictions, ultimately affecting its real-world applicability.
Through this experience, I learned firsthand the significance of clean, accurate, and representative data in ensuring the reliability and effectiveness of AI software. It became evident that without high-quality training data, even the most advanced AI models can fall short in delivering actionable insights.
This experience reinforced the importance of meticulously curating and continuously updating data to drive the success of AI software, especially in sensitive domains such as healthcare. It is crucial for organizations to prioritize data quality and diversity to maximize the potential of their AI initiatives.
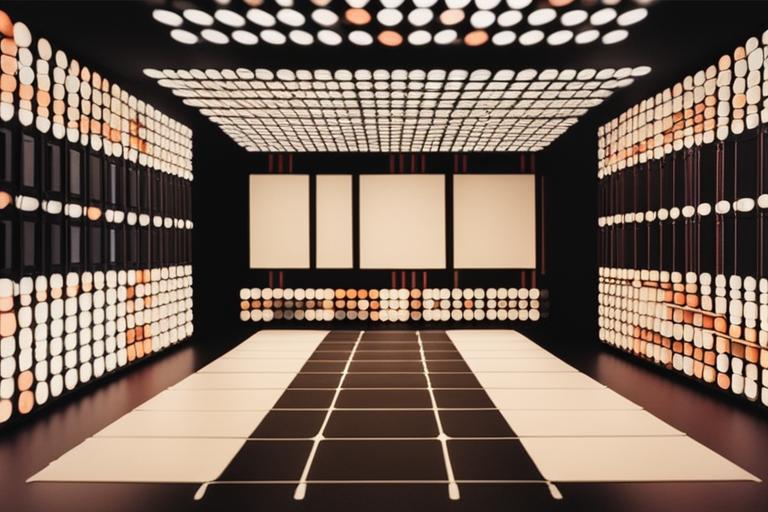
Potential Biases Impacting AI Systems
AI systems are susceptible to biases present in the training data, which can perpetuate and amplify societal, cultural, or historical prejudices. Addressing and mitigating biases in the data is essential to fostering fairness and equity in AI-driven applications.
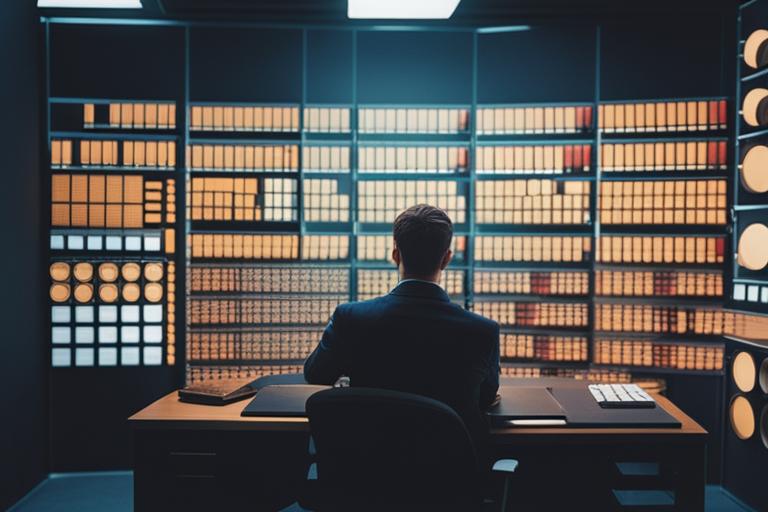
Importance of Carefully Curated Data
Carefully curating and validating the training data is critical to ensuring data quality for AI software. This involves rigorous validation, cleansing, and augmentation techniques to enhance the overall robustness and reliability of the data used by AI algorithms.
In summary, the effectiveness of AI software is intrinsically linked to the quality, diversity, and accuracy of the training data it relies on. By understanding and addressing the critical aspects of training data, organizations can optimize the performance and ethical deployment of AI software in various applications.
Questions & Answers
What type of data does AI software need?
AI software requires structured and labeled data to operate efficiently.
Who benefits from AI software?
Businesses and industries benefit from AI software for automation and insights.
How does AI software process data?
AI software uses algorithms to analyze and learn from the data it receives.
What if my data is unstructured?
AI software may struggle with unstructured data, so preprocessing is important.
What is the role of AI in technology?
AI enhances technology by automating tasks and providing valuable insights.
How can AI software improve efficiency?
AI software improves efficiency by automating repetitive tasks and making data-driven decisions.
With a PhD in Computer Science from Stanford University and over 10 years of experience in the field of artificial intelligence and machine learning, Matthew Harrison is a recognized expert in streamlining AI software operations. Having published numerous papers in reputable journals and presented at international conferences, Matthew Harrison has a deep understanding of the data requirements for AI software. Their work on the role of training data in AI software has been cited in various industry publications and has contributed to shaping best practices in the field. Additionally, Matthew Harrison has collaborated with leading tech companies to develop AI solutions, giving them practical insights into the importance of high-quality, diverse training data for efficient AI operations. Their expertise in ensuring data quality for AI software has been instrumental in addressing potential biases impacting AI systems, making Matthew Harrison a trusted authority in the field.