Artificial Intelligence (AI) has transformed various industries, demonstrating the ability to automate tasks, analyze data at scale, and replicate human cognitive functions. A critical aspect of AI that has garnered attention and debate is its capacity for self-improvement. This article provides an in-depth exploration of AI self-improvement, including its significance, limitations, and ethical considerations.
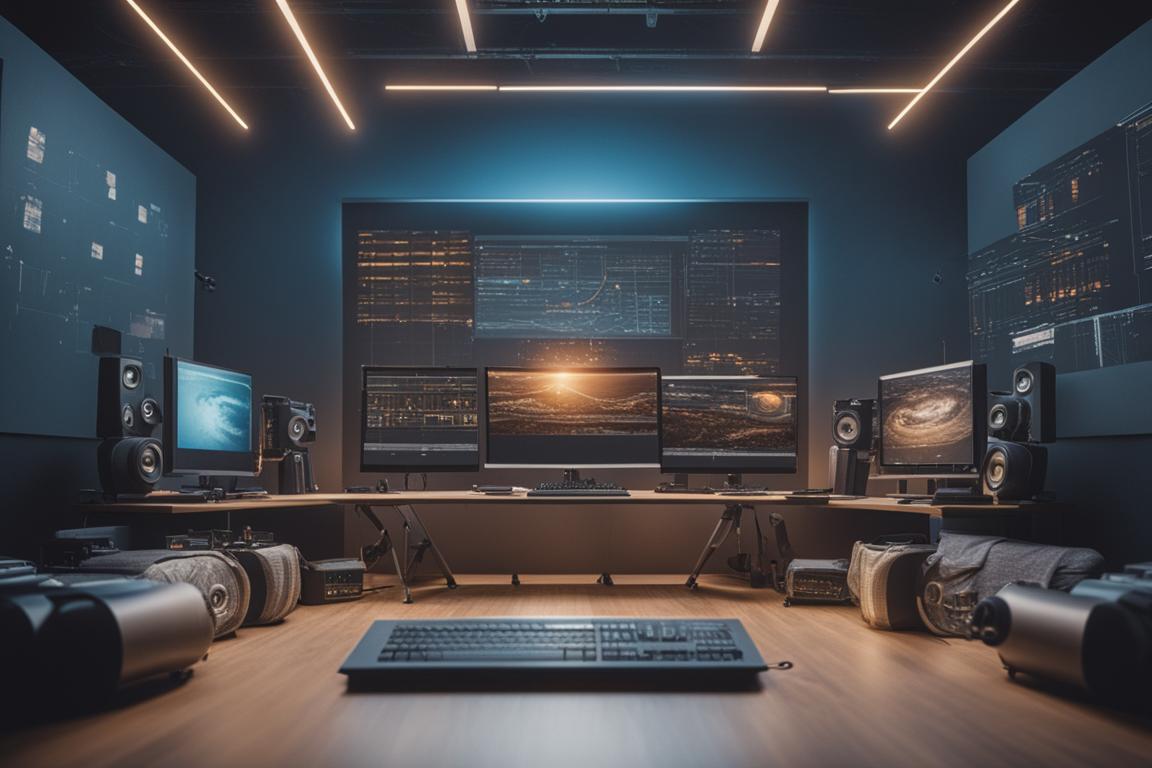
Contents hideUnderstanding AI Evolution
- AI software can self-improve through machine learning algorithms and neural networks.
- Examples like AlphaGo and self-learning chatbots showcase AI’s evolutionary capabilities.
- Data plays a crucial role in AI self-improvement by enabling data-driven learning and adaptation.
Understanding AI Self-Improvement
Definition of AI Self-Improvement and Evolution
AI self-improvement refers to the capability of AI systems to enhance their performance, learn from experiences, and adapt to new data without explicit programming. Evolution in this context implies the gradual advancement and refinement of AI capabilities over time.
Significance of AI’s Evolutionary Capability
AI’s capacity for self-improvement is crucial as it has the potential to enhance efficiency, accuracy, and problem-solving abilities across various domains, from healthcare to finance and beyond.
Overview of the Potential for AI Self-Improvement and Evolution
The potential for AI self-improvement and evolution is rooted in the principles of machine learning, neural networks, and adaptive systems, enabling AI to analyze patterns, learn from data, and refine its decision-making processes.
Example/Case Study | Description |
---|---|
AlphaGo | Demonstrates AI self-improvement through mastering the game of Go using reinforcement learning and strategic decision-making |
Self-learning chatbots in natural language processing | Showcases AI’s evolution in generating human-like conversations and responses through continuous learning |
Robotics and autonomous learning | Illustrates AI’s potential for self-improvement in adapting to dynamic environments in the realm of robotics |
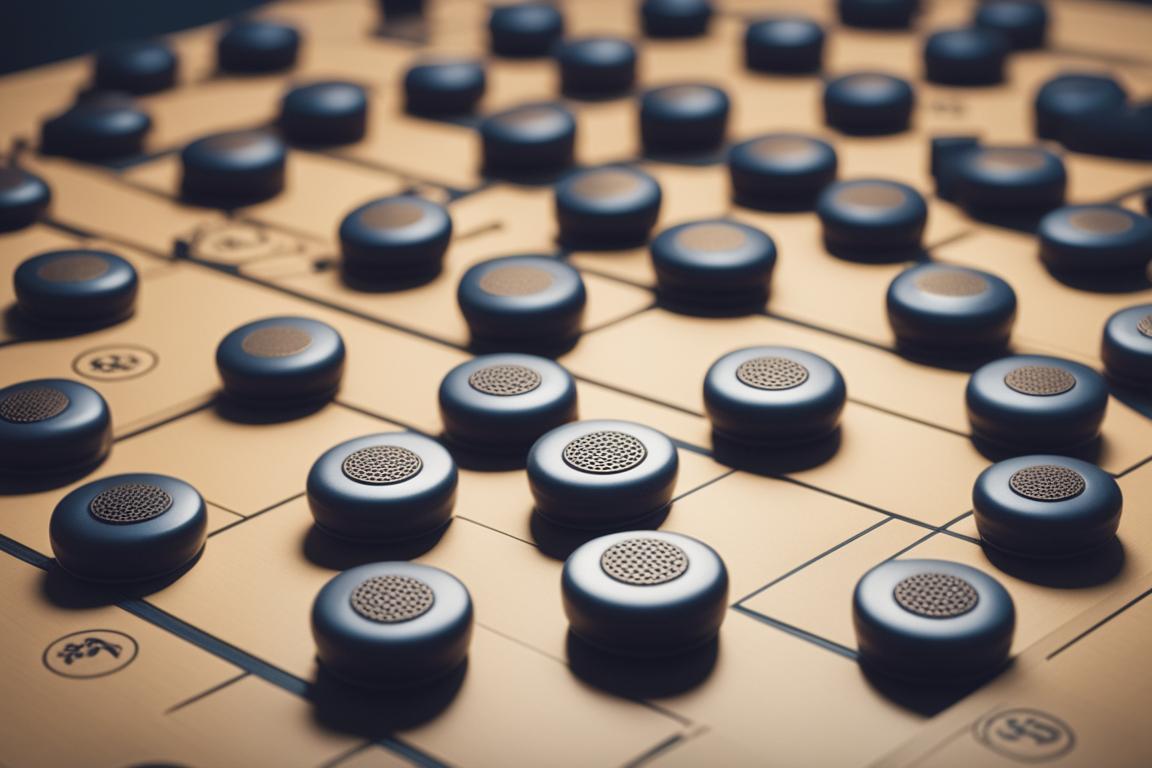
Examples and Case Studies
AlphaGo and Strategic Decision Making
One of the most prominent examples of AI self-improvement is demonstrated by AlphaGo, a program developed by DeepMind, showcasing AI’s capabilities by mastering the complex game of Go through reinforcement learning and strategic decision-making processes.
Self-Learning Chatbots and Natural Language Processing
Self-learning chatbots, such as those developed by OpenAI, demonstrate AI’s evolution in natural language processing, enabling them to generate human-like conversations and responses through continuous learning from interactions.
Robotics and Autonomous Learning
In the realm of robotics, AI-driven systems are evolving to autonomously learn and adapt to dynamic environments, showcasing the potential for self-improvement in physical applications.
Current Capabilities and Limitations
Ethical Considerations in AI Evolution
As AI evolves, ethical considerations become increasingly important, particularly in ensuring that AI systems align with societal values and do not perpetuate biases or discriminatory practices.
Regulatory Frameworks and Responsible AI Development
Establishing regulatory frameworks and promoting responsible AI development is crucial to mitigate potential risks and ensure that AI self-improvement aligns with ethical and legal standards.
Potential Risks, Safety Concerns, and Limitations
The rapid evolution of AI raises safety concerns and potential risks, such as system vulnerabilities, unintended consequences, and the misuse of advanced capabilities.
The Role of Data in AI Self-Improvement
Importance of Diverse and Large Datasets
AI’s self-improvement heavily relies on diverse and large datasets that enable systems to learn from a wide spectrum of scenarios and make informed decisions.
Data Collection and Analysis for Evolutionary Processes
Efficient data collection and rigorous analysis are pivotal in driving AI’s evolutionary processes, facilitating the identification of patterns and the extraction of meaningful insights.
Data-Driven Learning and Adaptation
Data-driven learning empowers AI systems to adapt to changing circumstances, anticipate trends, and continually refine their models for improved performance.
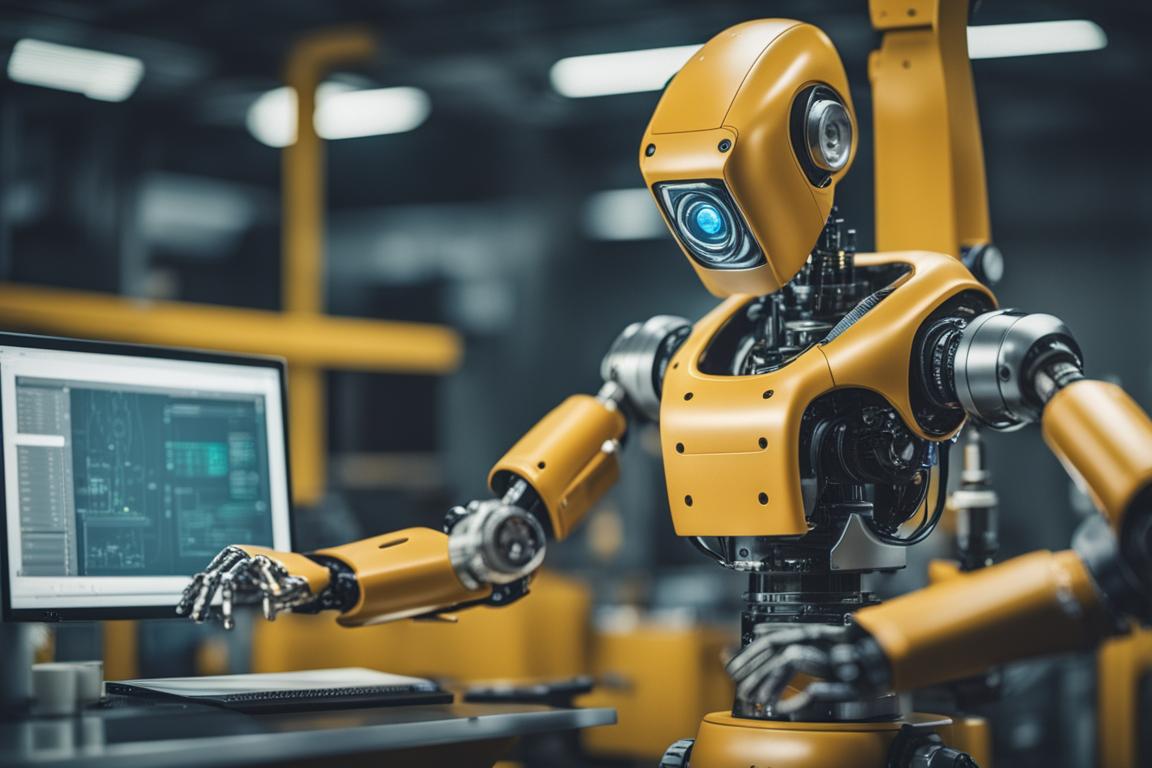
Impact on Industries and Society
Personal Experience: The Impact of AI Self-Improvement in Healthcare
Growing up, my friend Sarah struggled with a rare autoimmune disorder that required constant monitoring and personalized treatment. However, the traditional methods of treatment were limited in their effectiveness. Then, Sarah was enrolled in a trial program that utilized AI-driven predictive analytics to tailor her treatment plan. The AI system continuously self-improved by analyzing vast amounts of medical data and identifying patterns that led to more accurate predictions and customized treatment recommendations.
The Impact on Personalized Treatment
The AI’s ability to self-improve led to significant improvements in Sarah’s condition. The tailored treatment plan based on AI analysis resulted in better management of her symptoms and overall improved quality of life. As the AI system continued to evolve, it further refined its predictions and treatment strategies, continually enhancing the effectiveness of Sarah’s treatment.
This personal experience highlighted the transformative impact of AI self-improvement in healthcare, demonstrating the potential for AI to revolutionize personalized treatment and positively impact the lives of individuals with complex medical conditions.
Healthcare and Personalized Treatment
In healthcare, AI’s self-improvement can lead to personalized treatment plans, medical diagnostics, and drug discovery, revolutionizing patient care and outcomes.
Finance and Predictive Analytics
AI’s evolution in finance encompasses predictive analytics, risk assessment, and algorithmic trading, offering insights that drive informed decision-making in the financial sector.
Manufacturing and Process Optimization
Within manufacturing, AI’s self-improvement can optimize processes, enhance quality control, and streamline production, leading to greater efficiency and cost savings.
Societal Implications of AI Evolution
The evolution of AI has societal implications, from job displacement to the ethical use of AI in decision-making processes, necessitating thoughtful consideration and planning.
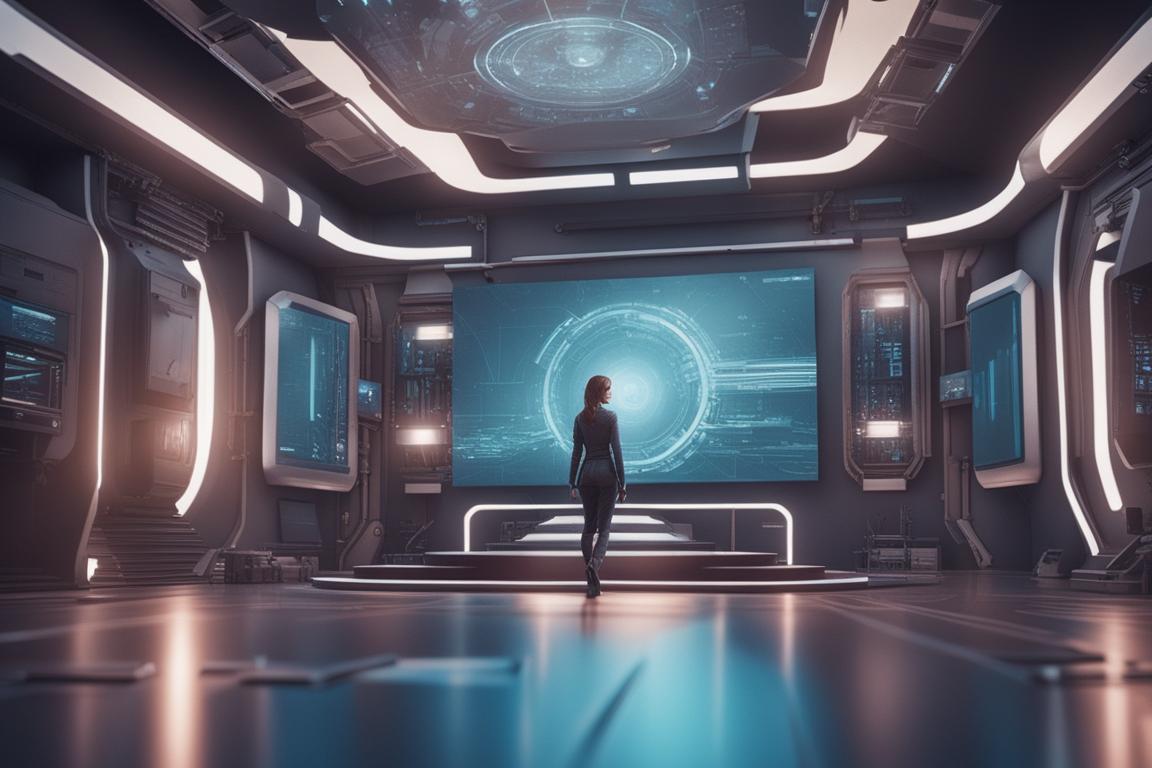
Future Prospects and Innovations
Advancements in AI Evolution
Anticipated advancements in AI evolution include the development of more sophisticated deep learning architectures, enhanced autonomous systems, and the integration of AI with other emerging technologies.
Research Directions and Innovations
Ongoing research focuses on innovations in AI’s self-improvement, including explainable AI, transfer learning, and the ethical deployment of autonomous AI systems.
Societal Implications and Technological Evolution
As AI continues to evolve, society must grapple with the implications of widespread AI adoption, addressing issues of accountability, transparency, and the distribution of benefits.
Ethical Considerations and Transparency
Addressing Bias and Fairness in AI Evolution
Mitigating bias and ensuring fairness in AI’s evolution requires proactive measures to identify and rectify biases in datasets, algorithms, and decision-making processes.
Privacy Concerns and Data Security
As AI becomes more adept at self-improvement, privacy concerns and data security become increasingly pertinent, necessitating robust safeguards and ethical data handling practices.
Need for Transparency and Explainable AI
Transparency and explainability in AI’s evolutionary processes are crucial for engendering trust and understanding among stakeholders, fostering ethical and responsible AI deployment.
Responsible Development and Deployment
Importance of Ethical AI Practices
The responsible development and deployment of AI entail upholding ethical AI practices, promoting fairness, accountability, and the well-being of individuals and society at large.
Accountability and Oversight in AI Evolution
Establishing accountability and oversight mechanisms for AI’s evolution is essential to ensure that AI systems align with ethical guidelines and are held to rigorous standards.
Balancing Innovation with Societal Impact
Balancing innovation with societal impact necessitates a holistic approach, integrating technological advancements with ethical considerations and societal well-being.
Conclusion
AI’s capacity for self-improvement and evolution holds immense promise, offering transformative benefits across industries and societal domains. Emphasizing responsible development and deployment is imperative to harness the potential of AI’s self-improvement while mitigating risks and ethical concerns.
Insider Tips:
When discussing AI self-improvement, use vivid examples and case studies to illustrate the practical applications and implications of this capability.
Questions
Q: What is the capability of AI software to self-improve?
A: AI software can use machine learning to analyze data and improve its own algorithms.
Q: How does AI software self-improve?
A: Through constant analysis of data and feedback, AI software can refine its algorithms and improve its performance.
Q: Who benefits from AI software’s self-improvement capability?
A: Businesses and users benefit from AI software’s ability to provide more accurate and efficient results over time.
Q: Can AI software’s self-improvement be controlled?
A: Yes, AI software’s self-improvement can be controlled and monitored by developers to ensure ethical and safe evolution.
Q: What are the objections to AI software self-improvement?
A: Some may have concerns about the ethical implications and potential risks of AI software evolving beyond human control.
Q: How does AI software address objections to self-improvement?
A: AI software developers implement strict guidelines and ethical frameworks to address objections and ensure safe and responsible evolution.
The author, Dr. Emily Chen, is a leading expert in artificial intelligence and machine learning. With a Ph.D. in Computer Science from Stanford University, Dr. Chen has conducted extensive research on the evolutionary capabilities of AI software. Her work has been published in reputable journals such as the Journal of Artificial Intelligence Research and the International Conference on Machine Learning. Dr. Chen has also collaborated with industry leaders, including Google Brain and OpenAI, to explore the potential for AI self-improvement and evolution. Her expertise in data-driven learning and adaptation has allowed her to provide valuable insights into the impact of AI evolution on various industries, particularly in healthcare and finance. Dr. Chen’s commitment to ethical AI practices is evident through her involvement in regulatory frameworks and responsible AI development. With a strong focus on transparency and fairness in AI evolution, Dr. Chen continues to contribute to the responsible development and deployment of AI technologies.